Overview
The Power of Perception: Why Public Image Matters
In today's world, how we are perceived by others can significantly impact our success. This is especially true for public figures, from politicians and CEOs to celebrities and athletes. Their personalities play a major role in how the public views them, which can influence everything from elections and brand deals to approval ratings and popularity.
Traditionally, understanding public perception relied on surveys and focus groups, which can be expensive and time-consuming. But new research suggests there might be a faster, more efficient way: artificial intelligence.
Reading Between the Lines: How AI Understands Public Perception
Large language models (LLMs) like GPT-3 are trained on massive amounts of text data, including news articles, social media posts, and even Wikipedia entries. This data can reveal a lot about how people perceive public figures. By analyzing the way words are used in relation to a particular person's name, AI can start to identify patterns and associations.
For example, if articles about a politician consistently use terms like "strong" and "decisive," the AI might infer that this politician is perceived as someone who takes charge.
The Science Behind the Hype: Can Machines Really Judge Personality?
A recent study published in Nature's Scientific Reports explored this very question. Researchers found that GPT-3 could accurately predict how people perceived the Big Five personality traits (openness, conscientiousness, extraversion, agreeableness, and neuroticism) of public figures, simply by analyzing the location of their names within the machine's semantic space.
This suggests that AI models are not just picking up on basic public sentiment (like whether someone is liked or disliked) but can also grasp more nuanced aspects of personality.
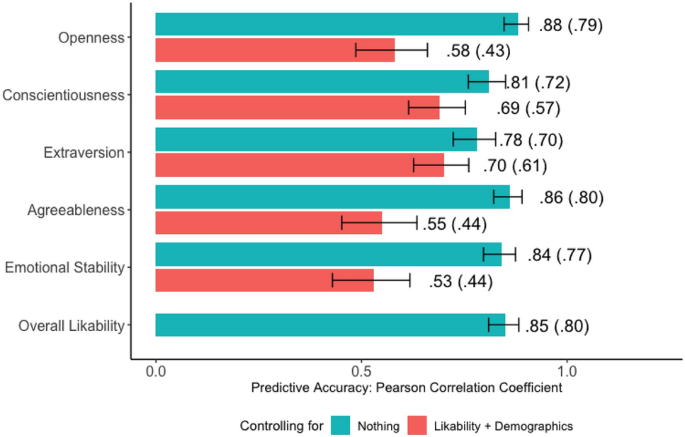
Friend or Foe? The Potential Benefits and Risks of AI Personality Prediction
The ability to predict public perception through AI has exciting possibilities. It could help public figures tailor their messages and communication styles to better resonate with their audience. For example, a politician might use AI insights to identify which personality traits are most valued by their voters.
However, there are also potential risks. Could this technology be used to manipulate public opinion? And how will it affect the way we perceive public figures themselves, if we know a machine can predict how others see them?
The Future of Perception: What This Means for Public Figures and Us
The use of AI in understanding public perception is still in its early stages. But it's a development worth watching. As AI continues to evolve, it will be interesting to see how it shapes the way we interact with and understand public figures, and perhaps even ourselves.
Summary of the Nature Study
Large language models like GPT-3 can accurately predict how the general public perceives the personality traits of famous individuals based solely on the semantic relationships between the individuals' names within the model's vast linguistic space.
Research Question
Can large language models (LLMs) predict how people perceive the personalities of public figures?
This question is important because understanding public perception is crucial for many public figures, and traditionally gauging this perception is expensive and time-consuming.
Methodology
- Researchers used a large language model, GPT-3, to analyze the location of public figures' names within its semantic space.
- Researchers collected personality ratings of 226 public figures from 600 human raters on the Big Five traits (openness, conscientiousness, extraversion, agreeableness, emotional stability) and general likability.
- Using cross-validated linear regression, the study predicted human perceptions from the location of public figures' names in GPT-3's 12,288-dimensional semantic space.
- Prediction accuracy ranged from Pearson r=.78 to .88 across the Big Five traits before controlling for likability/demographics, and r=.53 to .70 after controls, representing huge effect sizes.
- Human raters then provided their perceptions of the Big Five personality traits (openness, conscientiousness, extraversion, agreeableness, and neuroticism) and likability of 226 public figures.
- A statistical method (cross-validated linear regression) was used to assess how well the location of a public figure's name in GPT-3's semantic space predicted the human raters' perceptions.
Human participants were recruited through the Prolific platform to rate each public figure's likeness and Big Five personality traits (openness, conscientiousness, extraversion, agreeableness, and neuroticism). These ratings were then compared to the semantic relationships between the public figures' names within GPT-3's linguistic space using advanced machine learning techniques.
The results of the study demonstrated a remarkable level of accuracy in predicting human perceptions of public figures' personalities solely based on their names' locations within the LLM's semantic space. This finding highlights the immense potential for AI to uncover hidden patterns and insights within vast amounts of linguistic data, offering new avenues for research and practical applications in various domains.
Results
- The study found that GPT-3's location of public figures' names could predict human-rated personality traits and likability with high accuracy (ranging from r = .78 to .88 without controls and from r = .53 to .70 when controlling for demographics and likability).
- Accuracy exceeded the predictive power of individual human raters and was higher for more popular figures. Models showed strong face validity based on the personality-descriptive adjectives at the extremes of predictions.
- Findings expand our understanding of the rich information, including perceptions of individual traits, encoded in word embeddings from large language models.
Far-Reaching Implications for Various Disciplines
- This research suggests that LLMs like GPT-3 can capture signals related to people's personalities and how they are perceived by others.
- This opens up new possibilities for understanding public perception and potentially for public figures to tailor their communication strategies.
- It also raises questions about the potential misuse of such technology and the need for further research on how AI can be used to understand and influence perception.
- The ability to automatically infer perceived personality has implications across disciplines like political psychology, organizational behavior, and marketing. It also highlights potential privacy issues.
- Future directions include predicting personalities of figures absent from training data, using adjective embeddings directly, accounting for perception changes over time, and integrating with other data modalities.
- This proof-of-concept study showcases the potential for harnessing large language models to gain unprecedented insights into personality perception at scale.
The findings of this study have far-reaching implications across multiple domains, including psychology, political science, marketing, and beyond. Understanding how public figures' personalities are perceived can provide valuable insights into:
- Electoral success and approval ratings for politicians
- Reputation management and financial performance for companies and their CEOs
- Brand endorsement effectiveness and consumer engagement for celebrities
- Popularity and resonance of musicians their audience
By harnessing the power of LLMs to predict these perceptions, researchers and practitioners in various fields can gain a deeper understanding of the factors influencing public opinion and make more informed decisions.
The Power of Perception: How Public Figures' Personalities Shape Their Success
In an increasingly media-driven world, the way a public figure's personality is perceived can have a profound impact on their success and influence. From politicians to CEOs, celebrities to musicians, the impression they make on the general public can shape the course of their careers and the outcomes of their endeavors.
The Political Arena: Perceived Personality and Electoral Success
In the political realm, a candidate's perceived personality can be a deciding factor in their electoral success. Voters often form opinions about a politician's character, trustworthiness, and competence based on their public persona. Studies have shown that traits such as warmth, authenticity, and decisive leadership can significantly influence a candidate's approval ratings and ultimately, their chances of winning an election.
Corporate Leadership: CEOs' Perceived Personalities and Company Performance
For chief executive officers (CEOs), their perceived personality can have a direct impact on their company's reputation and financial performance. A CEO who is seen as visionary, innovative, and ethical can inspire confidence among investors, employees, and customers. On the other hand, a CEO perceived as untrustworthy or incompetent can damage a company's brand and lead to declining market share and stock prices.
Celebrity Endorsements: The Role of Perceived Personality in Brand Recognition and Purchase Intent
In the world of celebrity endorsements, a star's perceived personality can greatly influence the effectiveness of their brand partnerships. Consumers often form emotional connections with celebrities they admire, and these positive associations can transfer to the products or services they endorse. A celebrity seen as genuine, relatable, and trustworthy can boost brand recognition and drive purchase intent among their fans.
Music Industry: Perceived Personality and Artist Popularity
For musicians, their personality can play a significant role in their popularity and resonance with audiences. Artists who are seen as authentic, innovative, and charismatic often develop strong fan bases and enjoy long-lasting in the music industry. A musician's perceived personality can also influence the genres they are associated with and the collaborations they attract.
Traditional Methods of Measuring Perceived Personality: Surveys and Their Limitations
Historically, the most common method for measuring public figures' perceived personalities has been through surveys of the general public. Researchers and pollsters would design questionnaires to gauge people's opinions on various personality traits and characteristics. However, this approach has several limitations:
- Time-consuming: Conducting large-scale surveys can be a lengthy process, from designing the questionnaire to collecting and analyzing the data.
- Costly: Surveys often require significant financial resources to cover expenses such as participant recruitment, incentives, and data processing.
- Limited sample size: Surveys can only reach a limited number of people, which may not always be representative of the broader population.
The Promise of Digital Language Analysis: Predicting Personality Perceptions from Online Data
The explosion of digital communication and the growing availability of large language models (LLMs) have opened up new possibilities for predicting personality perceptions. LLMs, such as GPT-3/4, are trained on massive text corpora, including news articles, social media posts, and online discussions. These models can analyze the language used in public discourse to identify patterns and sentiments related to public figures' perceived personalities.
By leveraging the power of LLMs and other advanced natural language processing techniques, researchers can now study personality perceptions on an unprecedented scale. This approach offers several advantages over traditional survey methods:
- Real-time insights: Digital language analysis can provide up-to-date insights into public figures' perceived personalities as events unfold and opinions evolve.
- Cost-effective: Analyzing existing online data is often more cost-effective than conducting large-scale surveys.
- Broader reach: Digital language analysis can capture the opinions of a wider and more diverse population, including those who may not typically participate in surveys.
As the field of digital language analysis continues to advance, it holds great promise for understanding and predicting how public figures' personalities are perceived by the general public. This knowledge can inform strategies for reputation management, brand partnerships, and public engagement across various domains.
The Current Study
Unraveling Personality Perceptions: A Groundbreaking Study Design
To investigate the potential of large language models (LLMs) in predicting public figures' perceived personalities, the researchers designed a comprehensive study that combined a diverse dataset, human ratings, and advanced machine learning techniques.
A Diverse Dataset: 226 Popular Public Figures from Pantheon 1.0
The study utilized a dataset of 226 popular public figures sourced from the Pantheon 1.0 project. This dataset was carefully curated to ensure a wide range of professions and domains were represented, including:
- Politics and government
- Business and entrepreneurship
- Entertainment and media
- Science and technology
- Sports and athletics
The selection criteria for these public figures were based on the number of Wikipedia page views they received, ensuring that the dataset included individuals with significant public attention and interest. Additionally, the dataset included demographic information, such as age and gender, to allow for further analysis and control of potential confounding variables.
Human Ratings: Insights from 600 Prolific Raters
To gather human perceptions of the public figures' personalities, the researchers collected ratings from 600 participants through the Prolific platform. Each participant was asked to rate a subset of the public figures on two key dimensions:
- Likability: Participants rated each figure's overall likability on a scale from highly unlikable to highly likable.
- Big Five Personality Traits: Participants assessed each figure's personality using the well-established Big Five framework, which includes openness, conscientiousness, extraversion, agreeableness, and neuroticism.
On average, each public figure received ratings from 18.89 participants, ensuring a robust and reliable measure of perceived personality. To maintain data quality, the researchers removed public figures who received insufficient ratings or were not familiar to enough participants.
Predicting Human Ratings: The Power of GPT-3 Embeddings
To harness the potential of LLMs in predicting perceived personalities, the researchers utilized GPT-3, a state-of-the-art language model trained on a vast corpus of online text. The key to this approach lies in the concept of semantic space – the high-dimensional representation of words and phrases within the model's architecture.
The researchers hypothesized that the location of a public figure's name within GPT-3's semantic space would contain valuable information about their perceived personality. To test this, they employed Ridge regression, a machine learning technique well-suited for analyzing high-dimensional data. This method allowed the researchers to identify patterns and relationships between the semantic space coordinates and the human personality ratings.
To ensure the robustness and generalizability of their findings, the researchers employed cross-validation techniques. This process involves repeatedly splitting the dataset into training and testing subsets, allowing the model to learn from a portion of the data and then validate its predictions on unseen examples. By using cross-validation, the researchers minimized the risk of overfitting and ensured that their results would hold up in real-world applications.
Assessing Prediction Accuracy: A Multi-Faceted Approach
To evaluate the performance of their personality perception prediction model, the researchers employed several complementary metrics:
- Raw Correlations: The researchers calculated the Pearson correlation coefficients between the model's predictions and the actual human ratings. These raw correlations provide a straightforward measure of the model's predictive power.
- Correction for Attenuation: To account for the inherent noise and variability in human ratings, the researchers applied a correction for attenuation based on the inter-rater reliability of the Prolific participants. This adjustment helps to estimate the model's true predictive capacity, as if it were predicting against a perfectly reliable set of human ratings.
- Benchmark Comparisons: To put their results into context, the researchers compared the model's predictive accuracy to established benchmarks in other domains, such as medical diagnostics. This comparison highlights the impressive performance of the LLM-based approach and underscores its potential for real-world applications.
By employing this multi-faceted approach to assessing prediction accuracy, the researchers provide a comprehensive and rigorous evaluation of their methodology, setting the stage for future applications and innovations in the field of personality perception research.
Results
Remarkable Accuracy in Predicting Perceived Personality from Name Embeddings
The results of the study demonstrated an extraordinary level of accuracy in predicting public figures' perceived personalities their name embeddings within GPT-3's semantic space. The Pearson correlation coefficients between the model's predictions and human ratings ranged from an impressive 0.78 to a staggering 0.88 across the Big Five personality traits.
These correlations translate to effect sizes that are considered "huge" in the field of psychological research, underscoring theustness and practical significance of the findings. Remarkably, the model's predictive power was found to be comparable to, or even superior to, the judgments made by individual human raters.
Popularity Matters: Higher Accuracy for Well-Known Public Figures
An intriguing finding from the study was that the model's predictive accuracy was even greater for more popular public figures. This suggests that the vast amount of online data available for these individuals allowed GPT-3 to form a more comprehensive and nuanced representation of their perceived personalities.
As public figures gain more attention and media coverage, the language model is able to capture a richer array of associations and contexts related to their names, leading to more accurate predictions of how they are perceived by the general public.
Face Validity: Meaningful Associations Between Traits and Public Figures
The researchers found strong evidence for the face validity of their approach by examining the public figures who were predicted to score highest and lowest on each personality trait. These associations aligned closely with common intuitions and observations about the individuals in question.
For, public figures predicted to be high in openness tended to be creative artists, such as musicians and actors, while those predicted to be low in openness were more likely to be conservative political leaders. The model also captured meaningful links between perceived personality and factors such as profession, gender, and overall likability.
Controlling for Confounds: Robust Predictions Beyond Demographics and Likability
To ensure that the model's predictive power was not simply a reflection of demographic factors or overall likability, the researchers conducted additional analyses controlling for these potential confounds. Impressively, the model's accuracy remained high even after accounting for variables such as age, gender, and general positive or negative sentiment toward each public figure.
The ability to predict perceived personality traits beyond what can be explained by demographics and likability suggests that the language model is capturing unique and valuable information about how individuals are perceived by the public. This level of predictive accuracy is comparable to that of established medical diagnostic tools, highlighting the potential for this approach to be applied in various domains.
Interpreting the Models: Personality-Descriptive Adjectives Align with Trait Definitions
To further validate and interpret the personality prediction models, the researchers examined the adjectives that were most strongly associated with each end of the trait spectra. They found that the adjectives at the extremes of the model's predictions closely matched the definitions and common descriptions of the Big Five traits.
For instance, adjectives such as "creative," "curious," and "adventurous" were associated with high openness, while "traditional," "conservative," and "conventional" were linked to low openness. This alignment between the model's predictions and established personality descriptors provides additional support for the validity of the approach.
Capturing Behavioral Correlates: Insights Beyond Self-Report
Interestingly, the models were able to capture meaningful behavioral correlates of personality traits, even though the training data did not explicitly include this information. For example, the adjective "alcoholic" was strongly associated with low conscientiousness, low agreeableness, and low emotional stability, aligning with well-established research findings on the links between personality and substance abuse.
This suggests that the language model is able to pick up on subtle cues and associations within the text data that reflect real-world patterns and behaviors. By leveraging the vast amount of information contained within its training corpus, GPT-3 can provide insights into perceived personality that go beyond what is typically captured by self-report measures.
Discussion
New Possibilities: Implications of LLMs for Personality Perception Research
The findings of this study have far-reaching implications for the field of personality perception and beyond. By demonstrating the ability of large language models (LLMs) to accurately predict how public figures' personalities are perceived, the researchers have opened up new avenues for studying and understanding this crucial aspect of social cognition.
LLMs as a Tool for Studying Personality Perception
One of the key implications of this research is that LLMs can serve as a powerful tool for studying personality perception on a large scale. By leveraging the vast amounts of text data available online, researchers can gain insights into how people form impressions of others' personalities based on the language used to describe them.
This approach offers several advantages over traditional methods, such as surveys or laboratory experiments, which are often limited in terms of sample size, diversity, and ecological validity. With LLMs, researchers can study personality perception in a more naturalistic context, capturing the complex and dynamic nature of this process as it unfolds in real-world settings.
Relevance Across Disciplines: From Political Psychology to Organizational Behavior
The implications of this research extend far beyond the domain of personality psychology. The ability to predict and understand how public figures' personalities are perceived has significant relevance for a wide range of disciplines, including:
- Political Psychology: Insights into how voters perceive the personalities of political candidates can inform campaign strategies and help predict electoral outcomes.
- Organizational Behavior: Understanding how employees, managers, and leaders are perceived by their colleagues and subordinates can shed light on important factors such as job satisfaction, team dynamics, and organizational culture.
- Marketing and Consumer Psychology: Knowledge of how celebrities and influencers are perceived by their followers can guide endorsement deals and help brands align with personalities that resonate with their target audiences.
By bridging the gap between these diverse fields, the current research highlights the interdisciplinary potential of LLMs for studying personality perception and its far-reaching consequences.
Expanding Our Understanding of Embeddings: Encoding Personality Information
The success of the current study in predicting personality perceptions from name embeddings also expands our understanding of what information these embeddings can encode. While previous research has shown that word embeddings can capture semantic relationships and cultural biases, the present findings suggest that they can also encode more nuanced and subjective aspects of meaning, such as personality traits.
This insight opens up new possibilities for exploring the richness and complexity of human language using computational methods. By examining the structure and content of embeddings, researchers may be able to uncover subtle patterns and associations that reflect the way people think about and perceive others' personalities.
Potential Extensions and Applications: Predicting Personality Beyond the Training Data
While the current study focused on predicting the perceived personalities of public figures included in the LLM's training data, the approach could potentially be extended to individuals who are not explicitly mentioned in the model's corpus. By analyzing the language used to describe a person in other contexts, such as social media posts or news articles, researchers could estimate their location in the semantic space and make inferences about their perceived personality.
Additionally, future research could explore the use of adjective embeddings as a way to reduce the need for human ratings in personality perception studies. By identifying the adjectives that are most strongly associated with each end of the personality trait spectra, researchers could potentially use these embeddings as a proxy for human judgments, saving time and resources.
Another important direction for future work is to account for changes in personality perceptions over time. As public figures' reputations and media coverage evolve, so too may the way their personalities are perceived by the general public. By incorporating temporal information into the modeling process, researchers could track these changes and gain insights into the dynamic nature of personality perception.
Privacy Considerations: Balancing Insights and Individual Rights
While the ability to predict personality perceptions from publicly available data offers valuable insights, it also raises important privacy considerations. The current study demonstrates the potential for LLMs to extract intimate information about individuals' perceived traits, even in the absence of explicit self-reports or behavioral data.
This raises questions about the extent to which such information should be considered private and protected, particularly in cases where the perceptions may not align with an individual's true personality. As research in this area advances, it will be crucial to develop ethical guidelines and best practices for balancing the benefits of personality perception research with the rights and privacy of individuals.
Perceived vs. Actual Traits: The Importance of Distinguishing Between Them
It is important to note that the current study focuses specifically on predicting perceived personality traits, rather than actual traits. While perceptions can have significant consequences for individuals and society, they may not always align with reality. People's judgments of others' personalities can be influenced by various biases, stereotypes, and contextual factors that may distort their impressions.
Therefore, when interpreting the results of this study and related research, it is crucial to distinguish between perceived and actual traits. While LLMs can provide valuable insights into how people are perceived by others, additional methods, such as self-reports or behavioral observations, may be needed to assess their true personalities accurately.
Limitations and Future Directions: Advancing the Science of Personality Perception
Despite the impressive results of the current study, there are several limitations and areas for future research to address. For example, the study focused on a relatively small sample of public figures, and it remains to be seen how well the approach would generalize to a broader and more diverse set of individuals.
Additionally, the study relied on a single LLM (GPT-3) and a specific set of personality traits (the Big Five). Future research could explore the use of other language models and personality frameworks to assess the robustness and generalizability of the findings.
Another important direction for future work is to examine the potential biases and limitations of using LLMs for personality perception research. Like any machine learning model, LLMs are only as unbiased as the data they are trained on, and they may perpetuate or amplify societal stereotypes and prejudices. Researchers must be mindful of these issues and work to develop methods for mitigating and correcting such biases.
Finally, future research could explore the integration of LLM-based personality perception with other methods and data sources, such as facial recognition, voice analysis, or social network analysis. By combining multiple modalities and approaches, researchers may be able to develop even more powerful and nuanced models of personality perception that capture the full complexity of this essential social-cognitive process.
But Wait! Our Students Have Seen This Before
The Power of Detailed Roles and Personas in Prompt Engineering
For readers of this publication and students of our Prompt Engineering Masterclass, the concept of providing a detailed role and persona to a large language model (LLM) might sound familiar. In fact, it's one of the foundational principles we've emphasized in our discussions on effective prompt engineering.
When working with LLMs, it's not enough to simply instruct them to "act as" a particular entity or character. To truly harness the power of these models and generate high-quality, contextually relevant responses, we need to go a step further. This is where the concept of Synthetic Interactive Persona Agents (SIPA) comes into play.

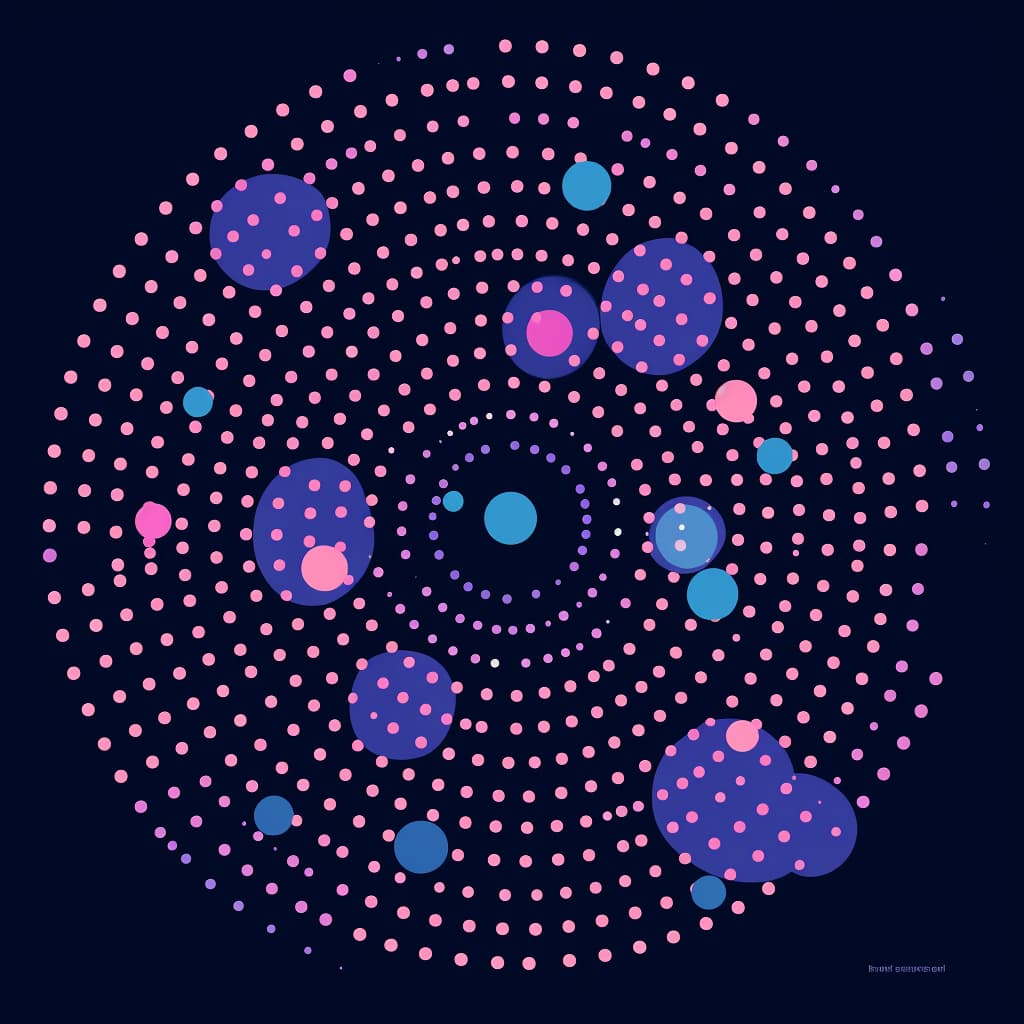
SIPA is a prompt engineering technique that involves creating detailed, well-defined roles and personas for the LLM to embody. By providing the model with a rich set of characteristics, background information, motivations, and even specific language patterns, we can guide it to generate responses that are not only accurate but also highly convincing and engaging.
Consider the difference between asking an LLM to "act as a customer service representative" versus providing it with a detailed persona: "You are a 35-year-old customer service representative named Emily, who has been working in the tech industry for 10 years. You are known for your patience, empathy, and ability to explain complex issues in simple terms. You have a calm demeanor and always strive to find the best solution for your customers."
By crafting such a detailed persona, we give the LLM a much clearer understanding of how it should respond and interact. This leads to more consistent, believable, and contextually appropriate responses, as the model has a well-defined framework to operate within.
The power of detailed roles and personas extends beyond customer service simulations. In the study on predicting perceived personalities using GPT-3 embeddings, we saw how the model was able to accurately capture and reflect human-like personality traits. By leveraging this ability and combining it with the SIPA approach, prompt engineers can create highly realistic and engaging AI-driven characters for a wide range of applications, such as virtual assistants, chatbots, educational tools, and even interactive storytelling.
Moreover, by using the insights gained from the study, prompt engineers can further refine their persona-building techniques. By incorporating personality-descriptive adjectives, contextual priming, and tone modulation, they can create even more nuanced and believable AI personas that closely mimic human behavior and perceptions.
In essence, the art of crafting detailed roles and personas lies at the heart of effective prompt engineering. By mastering this technique and combining it with the latest research insights, we can unlock the true potential of LLMs and create AI-driven interactions that are not only informative but also truly engaging and immersive.
A Number of Correlations with Prompt Engineering
The findings from the study on predicting public figures' perceived personalities using large language models (LLMs) like GPT-3 have several interesting correlations with the concept of Synthetic Interactive Persona Agents (SIPA) and the associated prompt engineering techniques.
- Simulating human-like perceptions: The study demonstrates that LLMs can accurately predict how humans perceive the personalities of public figures based on the semantic relationships between their names in the model's linguistic space. This aligns with SIPA's ability to generate synthetic data closely resembling human dialogues, behaviors, and attitudes. Both approaches highlight the potential for AI systems to simulate human-like perceptions and responses.
- Informing persona development: The study's methodology of using human ratings to train the LLM to predict perceived personalities could be applied to the development of SIPA personas. By collecting data on how humans perceive and respond to different personality types, researchers could use this information to create more accurate and realistic SIPA personas for various applications, such as customer service, market research, or political polling.
- Enhancing role-playing techniques: The role-playing approach in prompt involves the AI assuming various personas and responding according to assigned characteristics, attitudes, and behaviors. The study's findings suggest that LLMs can effectively capture and simulate human-like personality traits, which could be leveraged to create more convincing and dynamic SIPA personas for role-playing scenarios.
- Improving query reformulation: Query reformulation is a prompt engineering technique where the AI reformulates the original question into a more detailed and nuanced version aligned with its understanding. The study's approach of using personality-descriptive adjectives to interpret the LLM's predictions could be adapted to improve query reformulation in SIPA systems. By incorporating personality-related information into the reformulation process, SIPA could generate more contextually relevant and human-like responses.
- Multidisciplinary applications: The study's implications across various disciplines, such as political psychology, organizational behavior, and marketing, mirror the wide range of potential applications for SIPA technology. Both the study's findings and the SIPA use cases demonstrate the value of AI-driven insights into human behavior and perceptions for informing strategic decisions and improving human-computer interaction across diverse sectors.
By leveraging the ability of LLMs to capture human-like perceptions and traits, researchers and developers can create more accurate, convincing, and contextually relevant SIPA systems for a wide range of applications while remaining mindful of the associated ethical and privacy considerations.
Remarks
A Groundbreaking Study: Predicting Perceived Personality from Large Language Models
- By leveraging the vast amounts of text data encoded within GPT-3's semantic space, the researchers were able to accurately infer the perceived Big Five personality traits of a diverse set of individuals, with correlations ranging from 0.78 to 0.88.
- These findings further highlight the immense potential of LLMs as a tool for studying and understanding the complex processes underlying personality perception.
- The study's innovative approach, combining human ratings with advanced machine learning techniques, opens up new avenues for research and application across a wide range of disciplines, from psychology and sociology to marketing and political science.
Implications and Significance: Reshaping Our Understanding of Personality Perception
- The ability to predict and understand how public figures' personalities are perceived has significant real-world consequences, influencing everything from electoral outcomes and brand endorsements to organizational dynamics and social interactions.
- The findings suggest that LLMs can serve as a powerful complement to traditional methods, such as surveys and experiments, enabling researchers to gain insights into personality perception on an unprecedented scale and in a more naturalistic context.
- Moreover, the study's results shed new light on the richness and complexity of the information encoded within word embeddings. The fact that these embeddings can capture nuanced aspects of meaning, such as personality traits, highlights the immense potential of computational methods for exploring the structure and content of human language.
Future Directions: Advancing the Science of Personality Perception with LLMs
- One key area for exploration is the extension of the approach to individuals who are not explicitly mentioned in the LLM's training data. By analyzing the language used to describe a person in various contexts, researchers may be able to make inferences about their perceived personality based on their location in the semantic space.
- Methods for accounting for changes in personality perceptions over time. As public figures' reputations and media coverage evolve, so too may the way their personalities are perceived by the public. Incorporating temporal information into the modeling process could enable researchers to track these changes and gain insights into the dynamic nature of personality perception.
- Future research could explore the integration of LLM-based personality perception with other methods and data sources, such as facial recognition, voice analysis, or social network analysis. By combining multiple modalities and approaches, researchers may be able to develop even more powerful and nuanced models of personality perception that capture the full complexity of this essential social-cognitive process.
Ethical Considerations and Best Practices: Balancing Insights and Privacy
- The current study demonstrates the potential for these models to extract intimate information about individuals' perceived traits, raising important questions about privacy and consent. As research in this area advances, it will be crucial to address the ethical implications of using LLMs for personality perception.
- It will be necessary to develop guidelines and best practices for the use of LLMs in personality perception studies. This may involve establishing protocols for obtaining informed consent from participants, protecting individual privacy, and ensuring that the insights gained from these studies are used in a way that benefits society as a whole.
Continued Development of Persona in Agents in Prompt Engineering
As we've seen, crafting detailed roles and personas is a crucial aspect of effective prompt engineering when working with large language models (LLMs). However, creating a compelling persona is just the beginning. To truly leverage the potential of AI-driven agents and maintain engaging, contextually relevant interactions, we must focus on the continued development and refinement of these personas over time.
One key aspect of this ongoing development is adaptability. Just as humans learn, grow, and adapt based on their experiences and interactions, AI personas should be designed to evolve in response to user input and contextual changes. By incorporating feedback loops and dynamic updating mechanisms, prompt engineers can create agents that continuously improve their understanding of the user's needs, preferences, and communication style.
For example, consider a virtual assistant that is initially given a persona of a helpful, friendly, and professional aide. As the assistant interacts with users, it can gather data on the most common types of requests, the language patterns users employ, and even the emotional tone of the conversations. This information can then be used to refine the assistant's persona, making it more attuned to the specific needs and expectations of its user base.
Another important consideration in the continued development of AI personas is consistency. While adaptability is crucial, it's equally important to maintain a sense of coherence and continuity in the agent's persona. Inconsistencies in personality, knowledge, or communication style can quickly break the illusion of a believable, engaging AI entity.
To address this challenge, prompt engineers can leverage techniques such as memory mechanisms and context tracking. By equipping AI agents with the ability to remember and refer back to previous interactions, they can maintain a consistent narrative and build a more convincing sense of personality over time. Similarly, by tracking and incorporating contextual cues, agents can tailor their responses to the specific situation at hand, further enhancing the believability and relevance of their personas.
The continued development of persona in agents also involves a deep understanding of the target audience and the specific application domain. Prompt engineers must research and analyze user preferences, communication styles, and cultural norms to create personas that resonate with the intended users. This may involve collaboration with domain experts, user experience designers, and even psychologists to ensure that the AI personas are not only technically sound but also psychologically and emotionally engaging.
Moreover, as the field of prompt engineering continues to evolve, it's essential to stay up-to-date with the latest research and best practices. Studies like the one on predicting perceived personalities using GPT-3 embeddings offer valuable insights into the capabilities and potential applications of LLMs. By incorporating these findings into the persona development process, prompt engineers can create even more sophisticated and effective AI agents.
The continued development of persona in agents is a critical aspect of prompt engineering that requires ongoing attention, iteration, and refinement. By focusing on adaptability, consistency, audience understanding, and the incorporation of the latest research insights, prompt engineers can create AI personas that not only engage users in the moment but also build lasting, meaningful interactions over time.