Who would you pick to win in a head-to-head competition — a state-of-the-art AI agent or a mouse? This unexpected question serves as the starting point for an innovative study conducted by Isaac Kauvar, a Wu Tsai Neurosciences Institute postdoctoral scholar, and Chris Doyle, a machine learning researcher at Stanford. In a twist of outcomes, their research led to the development of a new AI training method, paving the path for more adaptive and flexible technologies in the future.
Exploring the Unexpected
To compare an AI agent and a mouse might seem like comparing apples and oranges. Yet, their study had a clear goal: to examine adaptability to new surroundings, a skill that animals are notably proficient in. In a seemingly simple experiment, Kauvar and Doyle placed a mouse and an AI agent in different environments with a red ball, aiming to measure who would explore the new object quicker. Can you guess the outcome?
Introducing Curious Replay
- Curious replay is a novel training method that stimulates AI agents to self-reflect on the most intriguing or novel occurrences they have recently come across.
- This method was designed based on observations of how animals naturally explore and adapt to their surroundings.
- This was demonstrated in an experiment involving a mouse and a simulated AI agent, both exposed to a new object - a red ball - in their respective environments.
- Interestingly, while the mouse quickly interacted with the ball, the AI agent failed to respond, highlighting gaps in the AI's performance.
- These insights led the team to use curiosity-inspired behaviors to enhance the AI system's abilities.
Enhancing AI Learning Through Curiosity
The central idea in this research is that curiosity plays a pivotal role in learning and adaptation, a concept that's as applicable to human beings as it is to AI.
- Curiosity encourages exploration and interaction with the surrounding environment, which is key to learning and adaptation.
- Kauvar and Doyle attempted to instil this element of curiosity in AI systems, urging the AI to 'reflect' on its most captivating or unusual experiences.
- This could potentially guide the AI's understanding of its environment and stimulate curiosity toward more elements, promoting further interaction and learning.
- The research team proposed that in a changing environment, it would be more productive for AI systems to prioritize replaying the most interesting experiences, rather than repeated exposures to the same.
Curious Replay in Action
Incorporating the concept of curious replay into the training of AI agents has shown immediate and encouraging results.
- Following the addition of the curious replay method, the AI agent started to interact more promptly with the novel object, in this case, the red ball.
- Further exploration involved applying curious replay to AI agents in the game of Crafter, a recognized test of creative problem-solving in AI.
- The agents had to learn survival and adaptation strategies, much like in the game Minecraft.
- With the incorporation of curious replay, there was a noticeable improvement in the performance of the AI agents, affirming the effectiveness of the method.
The Future Impact of Curious Replay
The successful application of curious replay in AI training opens up a world of potential for future AI research.
- The idea of creating AI agents that can efficiently adapt to new or changing environments by leveraging past experiences holds promise for more adaptable, flexible technologies.
- These could range from household robotics to personalized learning tools.
- Kauvar's research indicates that studying animal behavior could be a source of inspiration for enhancing AI systems further.
- In the broader context, this could aid in bridging the gap between AI research and neuroscience, potentially yielding insights into animal behavior and underlying neural processes.
Towards a Curious Future
The significant impact of curious replay in improving AI performance suggests the potential for its widespread use in future AI research. It paves the way for the development of much more adaptive and flexible technologies, such as personalized learning tools and household robotics. The application of curious replay in AI training makes a compelling case for the potential of animal-inspired learning methods to revolutionize AI systems.
Kauvar is enthusiastic about continuing to explore animal behavior as a source of inspiration for improving AI systems, and in the process, strengthen the bridge between AI research and neuroscience. Does this mean we might see a future where mice are the professors in AI development? While that's perhaps a stretch too far, the outcome of this study gives us cause to appreciate the humble mouse and recognize that we have much to learn from the creatures around us.
The moral of this tale? When it comes to exploring and adapting to the world around us, it appears curiosity, even in artificial intelligence, does more than kill the cat – it might just revolutionize the way AI learns.
Reference
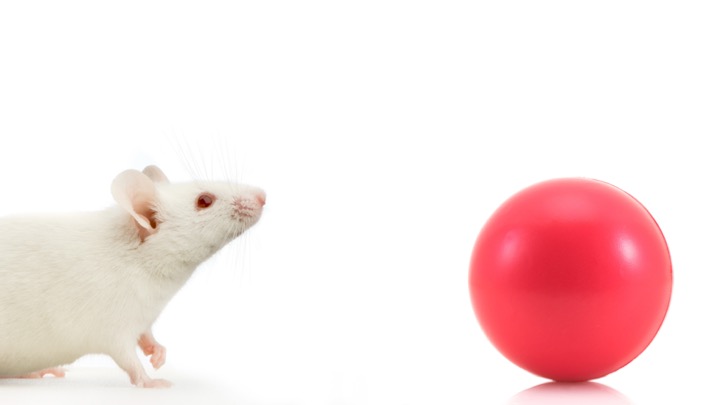